Excited to dive into the realm of artificial general intelligence (AGI) experiments, I’ve uncovered fascinating sparks that illuminate the early stages of this groundbreaking technology. In my exploration, I’ve encountered pioneering studies and innovative trials that hint at the immense potential of AGI to revolutionize various industries.
As I delve deeper into the world of AGI experimentation, I’m captivated by the creativity and ingenuity displayed by researchers pushing the boundaries of what’s possible. The sparks of AGI early experiments not only showcase the progress made in this field but also raise thought-provoking questions about the ethical and societal implications of advancing AI technologies.
Sparks of Artificial General Intelligence: Early Experiments With GPT-4
The Concept of AGI Versus AI
In my exploration of artificial general intelligence (AGI), I’ve encountered a crucial distinction between AGI and artificial intelligence (AI). While AI focuses on specific tasks and functions, AGI aims to mimic the breadth and depth of human cognitive abilities. This distinction sets AGI apart as a more comprehensive and versatile form of intelligence, capable of learning, reasoning, and problem-solving across a wide range of domains.
Key Features of AGI
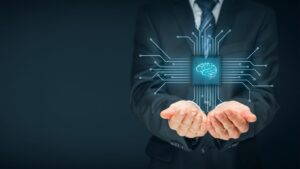
When delving into the realm of AGI, I’ve identified several key features that characterize this advanced form of intelligence. AGI exhibits adaptability, allowing machines to apply knowledge and skills across diverse scenarios without requiring reprogramming. Furthermore, AGI demonstrates creativity by generating novel solutions to complex problems, akin to human creative thinking. Its ability to generalize learning from one task to another showcases a level of cognitive flexibility that surpasses traditional AI systems. AGI’s potential to understand and learn from natural language and unstructured data marks a significant leap forward in bridging the gap between machines and human-like intelligence.
The Genesis of AGI: A Historical Overview
Pioneers in AGI Research
In tracing the genesis of Artificial General Intelligence (AGI), I recognize the pivotal role of pioneers in this field. These visionaries laid the groundwork for the transformative possibilities of AGI, propelling us towards a future where machines can exhibit cognition comparable to humans. Individuals like Marvin Minsky, John McCarthy, and Herbert Simon catalyzed the exploration of AGI, igniting a spark that continues to illuminate the path of AI development. Their early contributions set the stage for subsequent advancements, shaping the landscape of intelligent systems as we know it today.
Early Theoretical Foundations
Delving into the early theoretical foundations of AGI, I uncover a rich tapestry of ideas that underscore the complexity of replicating human-like intelligence. Theoretical frameworks such as symbolic AI, connectionism, and cognitive architectures served as pillars supporting the quest for AGI. These diverse approaches reflected researchers’ attempts to capture the essence of human cognition, paving the way for a multidimensional understanding of intelligence.
Breakthrough Experiments in AGI
Cognitive Architectures and Learning Algorithms
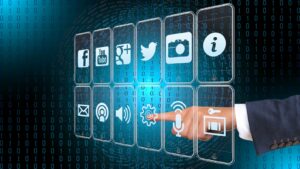
In the realm of artificial general intelligence (AGI), cognitive architectures play a pivotal role in simulating human-like cognitive functions. These architectures serve as blueprints for designing intelligent systems capable of reasoning, problem-solving, and learning. One prominent example is the Soar architecture, which models cognitive processes through the interaction of problem spaces, operators, and states.
Learning algorithms are essential components of AGI systems, enabling machines to acquire knowledge, adapt to new environments, and improve performance over time. Reinforcement learning, a fundamental algorithm in AGI, allows agents to learn through trial and error, receiving rewards for desirable actions. Bayesian networks and neural networks are also crucial in modeling uncertainty and processing complex patterns, respectively, enhancing the learning capabilities of AGI systems.
Embodied AGI and Early Robotic Systems
Embodied AGI emphasizes the integration of physical bodies with intelligent systems, enabling interaction with the environment and facilitating sensorimotor skills development. Early robotic systems such as Shakey the Robot and Cog exemplify the embodiment of AGI principles, manifesting autonomous decision-making and action execution in real-world scenarios.
Shakey the Robot, developed by Stanford Research Institute in the late 1960s, demonstrated rudimentary navigation and planning capabilities, marking a significant advancement in mobile robotics. Cog, a humanoid robot created by MIT in the late 1990s, showcased human-like communication skills and social interactions, paving the way for research in human-robot symbiosis.