In the ever-evolving domain of artificial intelligence, Retrieval-Augmented Generation (RAG) technology stands out as a significant advancement. By combining retrieval mechanisms with language generation, RAG AI enhances the contextual understanding and accuracy of AI systems, addressing the limitations of traditional models. For those new to this technology, a detailed exploration of what is RAG in AI can provide further insights which you can find in this article.
Understanding Retrieval-Augmented Generation (RAG)
RAG technology represents a paradigm shift in AI systems, emphasizing the importance of integrating vast knowledge bases with dynamic generation models.
What is RAG?
At its core, RAG combines two fundamental processes: information retrieval and language generation. Unlike traditional language models that rely solely on pre-trained data sets, RAG systems actively retrieve relevant information from external knowledge bases to generate responses. This mechanism allows RAG AI to overcome the static nature of conventional models, thereby addressing knowledge limitations effectively.
- Basic mechanism of retrieval and generation: RAG systems first identify relevant data through retrieval techniques before synthesizing this information into coherent responses.
- Difference from traditional language models: Traditional models are limited by their training data, while RAG dynamically accesses and utilizes current information.
- How RAG addresses knowledge limitations: By leveraging up-to-date data, RAG reduces inaccuracies and improves the relevance of AI-generated content.
Technical Architecture of RAG Systems
The architecture of RAG systems is intricate yet pivotal to their functionality.
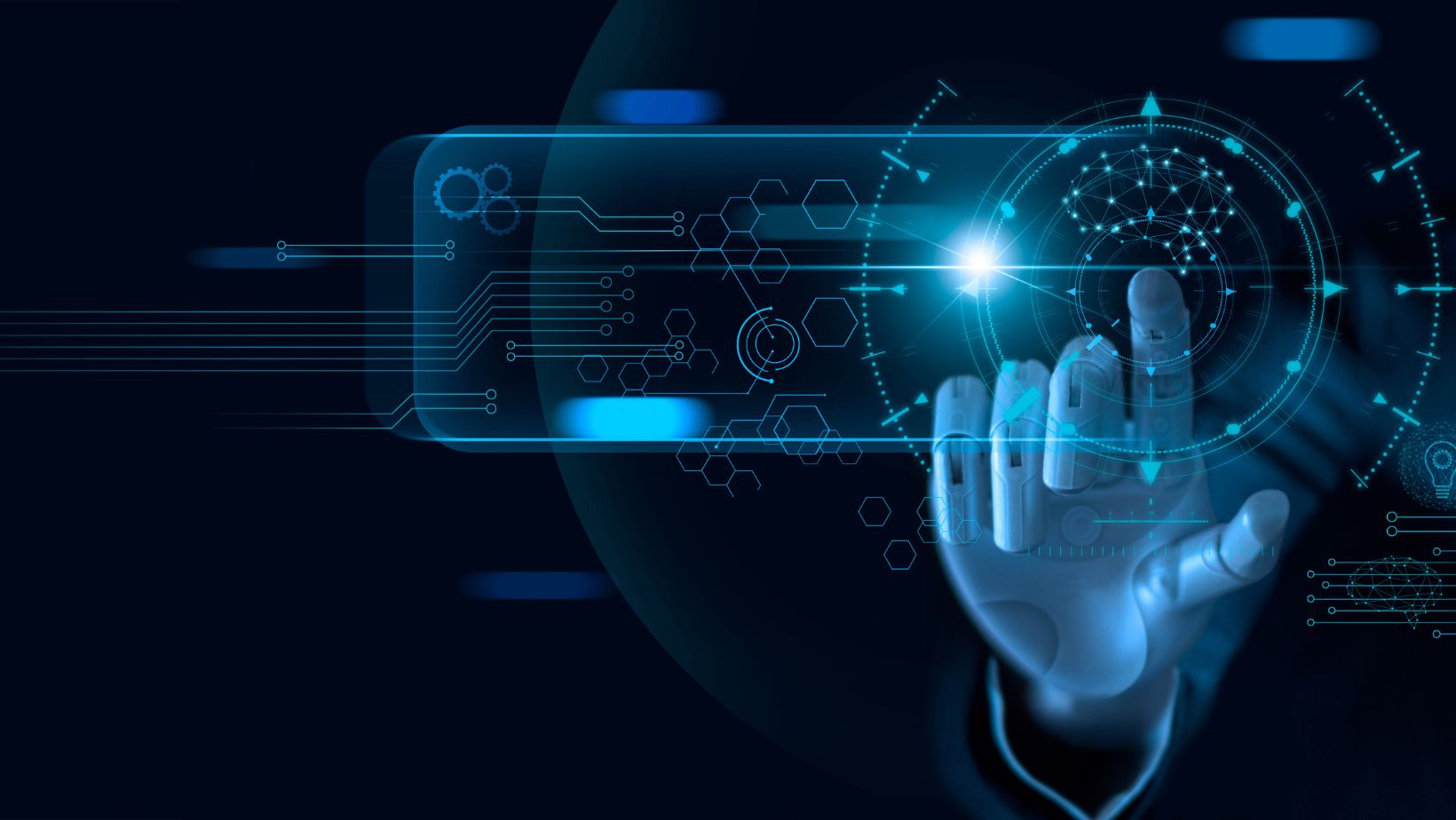
- Retrieval components: These are responsible for identifying and extracting pertinent data from vast databases. Efficient retrieval is vital for the timely and accurate performance of RAG systems.
- Knowledge base integration: Seamlessly integrating diverse data sources ensures that RAG AI can access a breadth of information, enhancing its contextual understanding.
- Generation and response synthesis: The retrieved data is then processed by sophisticated language models to generate accurate and contextually appropriate responses.
Key Benefits of RAG AI Technology
RAG technology offers myriad benefits, chiefly among them being the enhancement of accuracy and adaptability across various domains.
Accuracy and Precision
RAG AI excels in delivering precise and reliable information, reducing the common issue of AI hallucinations where models generate incorrect or nonsensical data.
- Reducing AI fabrication: By grounding responses in verifiable data, RAG minimizes the risk of generating false information.
- Real-time knowledge updating: Its ability to access the latest information ensures that RAG AI remains relevant and accurate.
- Verifiable information sources: Users can trace responses back to original data sources, fostering transparency and trust.
Practical Applications
The versatility of RAG AI is evident in its wide range of applications across different sectors.
- Enterprise knowledge management: By efficiently managing and retrieving organizational data, RAG AI optimizes decision-making processes.
- Customer support systems: RAG-driven systems offer enhanced customer interactions by providing accurate and contextually relevant responses.
- Research and academic applications: Researchers benefit from RAG’s ability to synthesize and present updated information, facilitating deeper insights and discoveries.
Implementation and Future of RAG
While RAG technology holds immense promise, its implementation presents certain challenges and opportunities for future advancements.
Technical Challenges
Several hurdles impede the seamless implementation of RAG systems, primarily concerning retrieval efficiency and scalability.
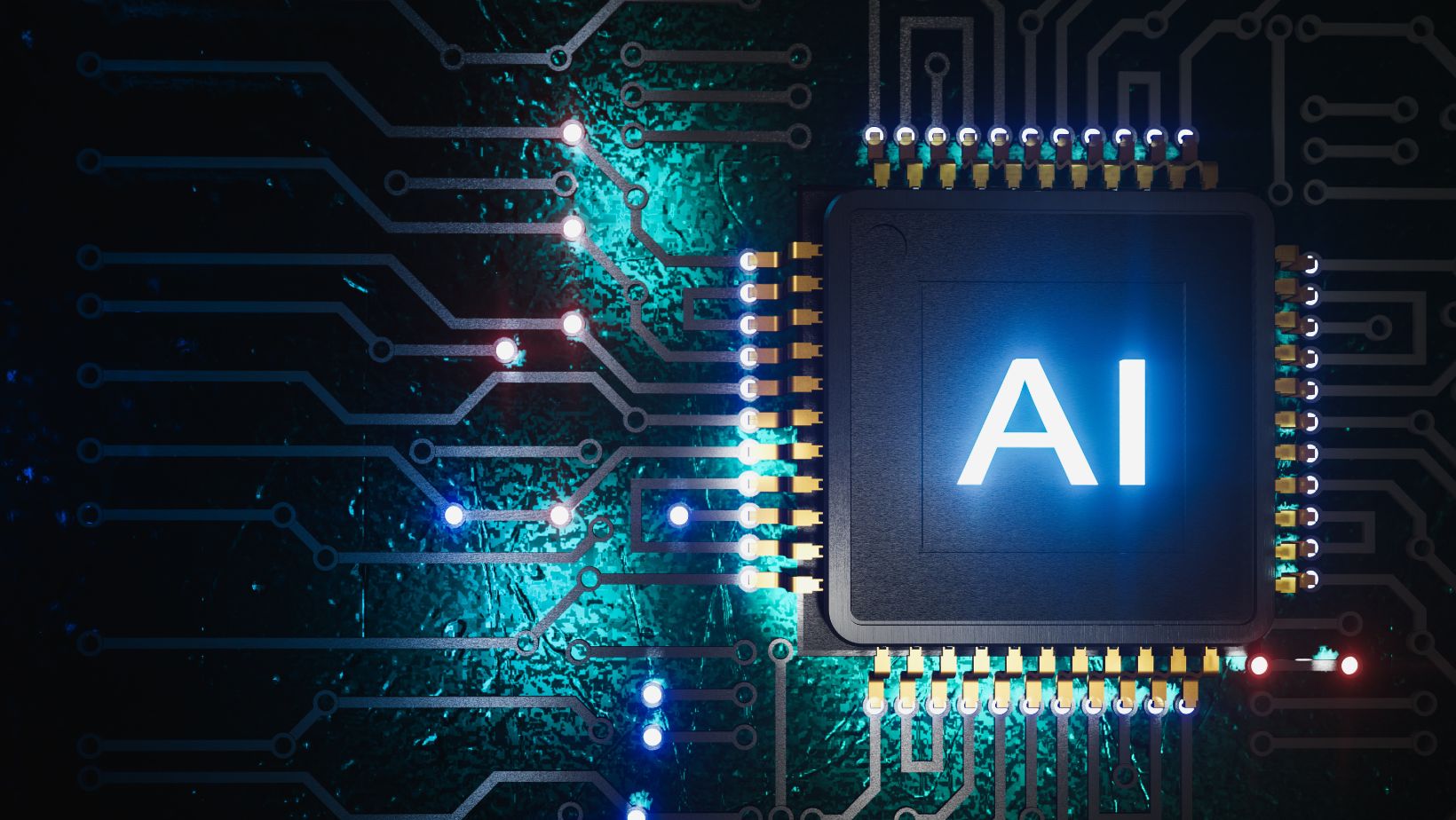
- Retrieval efficiency: Ensuring rapid access to relevant data without compromising accuracy is a key challenge for developers.
- Scalability of knowledge bases: As data volumes grow, maintaining scalable and efficient knowledge bases becomes imperative.
- Performance optimization: Balancing between computational efficiency and response accuracy is crucial for the practical deployment of RAG AI.
Future Outlook
The future of RAG AI is bright, with ongoing research paving the way for transformative changes across industries.
- AI research directions: Continuous innovations in machine learning and data retrieval promise to enhance RAG’s capabilities further.
- Potential breakthrough technologies: Advancements in quantum computing and neural network architectures could revolutionize RAG systems.
- Ethical considerations: As with all AI technologies, ethical implications surrounding data privacy and bias must be carefully addressed.
In conclusion, Retrieval-Augmented Generation technology is set to redefine the capabilities of AI systems. By enhancing contextual knowledge and precision, RAG AI not only improves accuracy but also expands the potential applications of AI across various domains. As the technology evolves, it promises to address existing challenges and open new avenues for innovation and transformation.